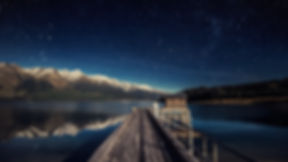
To justify the effectiveness of our proposed CLEVER model, we compared it with the following state-of-arts competitors. Moreover, here we list the brief description and reference of these models and release corresponding sample-code.
MMSC
This multi-modal spectral clustering method introduced in Heterogeneous image feature integration via multi-modal spectral clustering is to learn a commonly shared graph Laplacian matrix by unifying different views, where each modal stands for a type of feature from one single view.
RMKMC
The novel and robust multi-view k-means clustering method proposed in Multi-view k-means clustering on big data is able to uncover the consensus pattern and detect communities across multiple networks.
CoNMF
It is a co-regularized nonnegative matrix factorization model proposed in Comment-based Multi-View Clustering of Web 2.0 Items by extending NMF for multi-view clustering. It jointly factorizes the multiple matrices through co-regularization.