
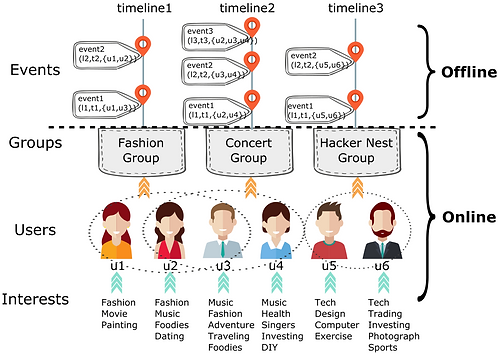
Event-based social networking service such as Meetup is capable of linking online virtual interactions and offline physical activities. Compared with the mono online social networking service (e.g., Facebook and Twitter), such dual-networks provide a complete picture of users’ online and offline behaviors that are, more often than not, compatible and complementary. In the light of this, we argue that joint learning over dual-networks offers us a better way to comprehensively understand user behaviors and their underlying organizational principles. Despite its value, few efforts have been dedicated to the following factors within a unified model: 1) local user contextualization, 2) global structure coherence, and 3) effectiveness evaluation. Towards this end, we propose a novel co-clustering model for community detection over dual-networks to jointly model the local consistency for a specific user and the global consistency of partitioning results across networks. We theoretically derived its solution. Besides, we verified our model in terms of multiple metrics from different aspects and applied it to the application of event attendance prediction.